Why we need more women in data science
By Lara Bogatu and Holly Silk on October 4, 2021 - 10 Minute ReadThe field of data science is booming. Data increasingly shapes every aspect of our lives and is being used across a variety of domains, from medicine to marketing.
Companies are now seeing the value of utilizing the data they hold to provide insights and create innovative solutions. This means that there’s an ever-increasing demand for data scientists – according to LinkedIn’s 2020 jobs on the rise report, the UK saw a 40% increase in AI positions. However, data science is currently very much a man’s world.
According to the same LinkedIn report, only 19% of those data science hires were women. As data becomes embedded in more and more aspects of our lives, we need more women to be involved in the design of the algorithms that will help to shape our future.
Even machines can be biased
Nowadays, algorithms can make suggestions on what to watch next on streaming apps, what could be of interest on a fashion retailer’s platform, make suggestions on university admissions or hiring, and will even predict if someone will recidivate or if they should be eligible to receive a bank loan. Some of these decisions are high-stake, and it’s paramount that they are made fairly – so that no person or group is favored or prejudiced.
(Un)fairness has been the subject of research for many works in recent years as, similarly to humans, algorithms can become biased and could yield unfair outputs. Unfairness can stem from a plethora of biases – let’s take a look at a few below!
Historical bias
Historical bias means the bias already exists and the socio-economic discrepancies can reflect into the data generation/aggregation process, even if there is a perfect feature selection. A 2018 study showed that the results of an image search for women who are CEOs showed rather few CEO women due to the fact that only 5% of Fortune 500 CEOs are women.
The algorithm was biased towards men because the data about men was more comprehensive than for women. Similarly, in another representative example, Amazon revealed that its recruiting tool showed bias against women. This happened because the machine learning models were trained on data from applicants by learning patterns in resumes submitted to the company over the past decade. Most applications were from men, thus showing a male dominance across the tech industry throughout the years. The same story is reflected in numerous similar articles and analyses that show the evolution of women in the STEM workforce.
Representation bias
This occurs when there is an issue with the way sampling is done and not all groups are equally represented – leading to underfit models for certain groups. For example, voice recognition is an important feature of the smart gadgets that surround us nowadays. In 2016, it was analyzed and it appeared that Google’s speech-recognition algorithm was 70% more likely to recognize a man’s speech than a woman’s.
This seemed to be a gender-biased algorithm against women, meaning that women were less efficient in their work if they were using this type of technology. However, in 2020, the study was rerun and it proved that, in noise-controlled environments, the bias disappears. However, the results of the 2016 study were not surprising as, more often than not, the training corpus may contain more data for the male, e.g. TIMIT contains 69% male entries.
Some decisions are high-stake, and it’s paramount that they are made fairly – so that no person or group is favored or prejudiced.
Evaluation bias
This stems from a (potentially) incomplete evaluation or from using inappropriate benchmarks. For example, a new Virtual Reality headset that was meant to track the eyes proved faulty when tested on most women. However, this was not the case if the tested subject was a man. After troubleshooting the issue, it appeared that the headset was not meant to work on eyes that wore makeup. After the headset was recalibrated, it worked as it was meant to.
Measurement bias
This is caused when an inappropriate measurement is used to assess a feature. This type of bias surfaced when evaluating the COMPAS tool, which was used to predict how likely someone is to recidivate based on prior arrests and friend and/or family arrests. According to the results, the rate of African-Americans who were predicted to recidivate, but didn’t, was higher (44.9%) than that of white people (23.5%). It turned out that minority communities are controlled more frequently by police so they have a higher number of arrests. Again, this is an example that shows the importance of having a diverse team (not necessarily from a gender point of view) as someone who is part of a certain community can potentially spot such issues more easily as they are directly involved.
Aggregation bias
This happens when incorrect conclusions are drawn for a subgroup, based on what was observed for other subgroups or when false assumptions about a population affect the outcome of the model. The following is not an AI-related example, however, it’s relevant for the topic: when assessing a car’s performance in a crash, i.e. the human damage, it was found that women are 47% more likely to be gravely injured and 17% more likely to die in a car crash compared to men.
This is due to the fact that most cars are tested on dummies that generalize over the whole population – and these dummies are more likely to have the characteristics of a man than those of a woman: 1.77m height, 76 kg weight. This poor generalization leads to cars passing the tests and being sold on the market, while being unsafe for a vast group of women (and men as well, but a potentially smaller group.) A second example, where AI would be used, would be in clinical trials where predicting a specific outcome for some human characteristics may not take into consideration the fact that the same characteristics may differ for different genders or ethnicities.
Although the list above is not exhaustive, it can be seen that there are several types of bias that can be caused mostly because either the training data is not representative enough for certain groups, e.g. gender, ethnic, racial minorities etc, or the evaluation is not suited for all groups, or that there is a societal bias that translates into the generated data – for instance, females in the STEM workforce. This may lead to bringing prejudice or causing unjust effects to these groups. However, some of them can be overcome by bringing diversity into the whole picture. If we have more inclusive teams we will discover more of the biases that algorithms display. This is the power of diversity.
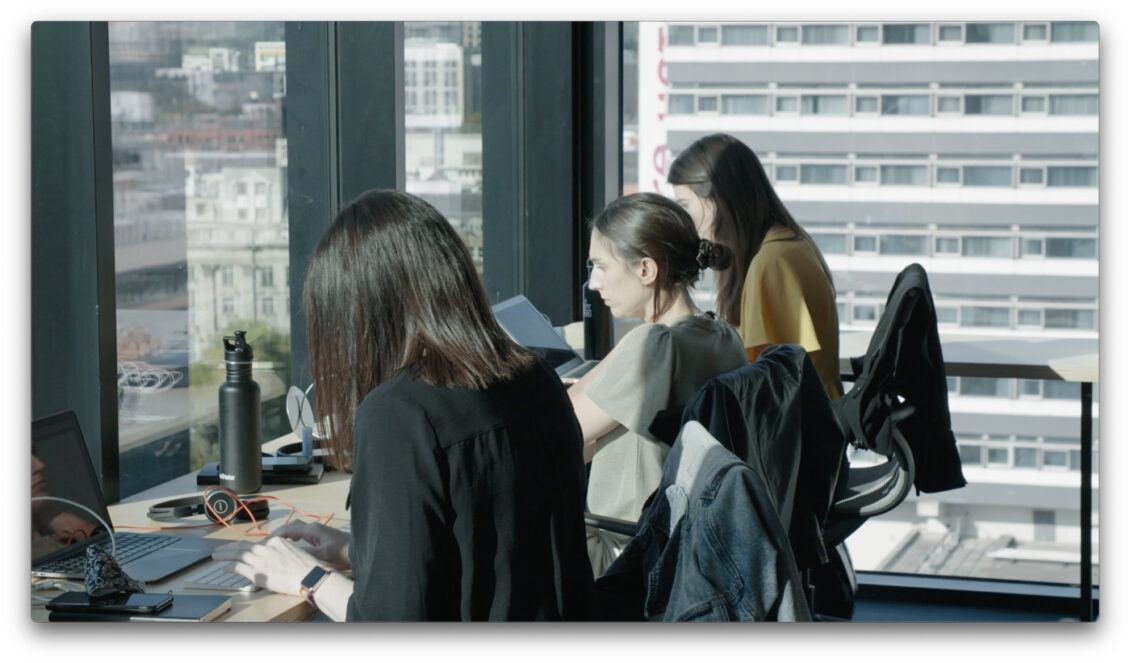
The power of diversity
Diverse teams are more likely to spot issues that might not be apparent to less diverse teams. Specifically, when people are included, they tend to surface problems that they face and that may not be visible to other groups. This also brings the perspective of a person from a minority group that may see opportunities where other people don’t.
As narrated in Sheryl Sandberg’s book, Lean in, when she became pregnant in 2014, she was working for Google, which was a well-established company at that time with a campus that needed a large parking lot. When it became difficult for her to travel through the parking lot due to her pregnancy, she went to one of the (male) founders and asked for pregnancy parking spots. The request was immediately approved and the founder admitted that he had never considered this.
This is a clear representation bias (a gap in the data) as no woman in a senior position (nor the male founders, obviously) became pregnant to experience the difficulties of walking through a large parking lot while having pregnancy side effects. A senior member had to become pregnant to make the change in the company; a change from which multiple women benefited afterwards at Google.
Diverse teams are more likely to spot issues that might not be apparent to less diverse teams. Specifically, when people are included, they tend to surface problems that they face and that may not be visible to other groups.
Some problems are never considered unless they have that correct standpoint, and that perspective may come solely from someone who is part of a minority group. If there is a lack of women within the field of data science, it increases the risk that data-driven solutions will be designed in ways that can overlook the interests of women. Increasing female representation is the only way to ensure that the priorities and points of view of women will be included in solutions as well as making sure women’s issues are considered in future research.
The benefits of diversity go further than just improving outcomes for women or those in minority groups. A more diverse team can improve the outcomes of a team, which in turn improves wider company performance. Having more women on a team has been linked with having higher levels of collective intelligence. More diverse teams consistently outperform even teams that are only made up of the highest ability members.
Teams with equal numbers of men and women are more likely to experiment, be creative and complete tasks. A team that has a variety of backgrounds and experiences is able to bring different perspectives and insights. This can widen the pool of knowledge and ideas leading to more creative and innovative solutions. Teams are stronger and achieve more if they are more diverse. If we want to build the best models we need to do it with a team that reflects the world around us.
Better team performance naturally leads to better company performance, and we see that in the results of companies whose make-up is more diverse. The most successful tech startups have two times as many women in senior positions when compared to less successful tech startups. Gender diversity has also been linked to increased sales, a bigger customer base and larger profits. Companies in the top quartile for gender diversity on executive teams are 25% more likely to have above-average profitability compared to companies in the fourth quartile.
As AI becomes embedded in more and more parts of our lives, we need to ensure that women are involved in the design of the models that will shape our future.
About Lara
Lara is a data scientist at Peak. She holds Bachelor and Master's degrees in general Computer Science and Software Engineering, and a PhD in data management from the University of Manchester. When she’s not working, Lara is either hanging out with friends, travelling the world or catching up with the latest reviews for newly-released gadgets.
About Holly
Holly is a data scientist at Peak, working on Demand Intelligence problems. She’s recently returned to Manchester where she studied for her undergraduate degree in Maths after completing her PhD at the University of Bristol. When not working you might find her running around like a headless chicken on the netball court or planning her next scuba diving adventure.
With credit to…
For some of the technical details, we used the following sources:
- N. Mehrabi, F. Morstatter, N. Saxena, K. Lerman, and A. Galstyan. 2021. A Survey on Bias and Fairness in Machine Learning. ACM Comput. Surv. 54, 6, Article 115 (July 2021), 35 pages.
- Criado-Perez, Caroline. 2019. Invisible women: data bias in a world designed for men.
More from Peak's data science team
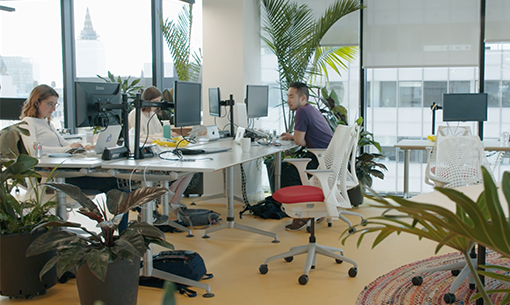
The importance of role models for women in data science
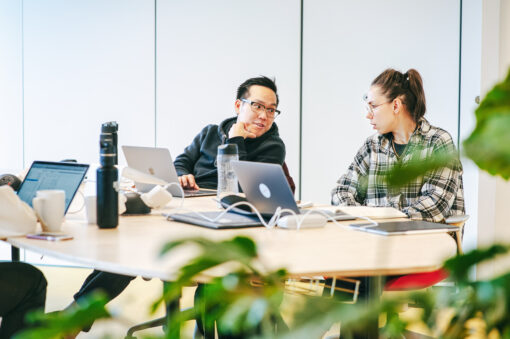