What is the best industry for data science?
By Chris Boddington on February 18, 2022 - 5 Minute ReadThe need for artificial intelligence (AI) and, more recently, Decision Intelligence (DI) has grown exponentially over the last few years.
Now, the prevalent view is that any business without it might not survive against their data driven competitors. The potential impact DI is expected to have on business results is so big, that Gartner forecasts over 33% of organizations will have data scientists building DI applications by 2023.
Whilst data science has existed in some of the biggest industries for a while now – such as finance, healthcare, entertainment and retail – it is increasingly used in more unexpected industries, such as the smart operation of machinery in agriculture and the preparation of fragrances. Not to mention the number of social and not-for-profit organizations out there using it to build a better world.
This is great news for data scientists, but as the reach of DI expands across businesses and the number of industries impacted by it, how do you decide which is the right industry for you as a data professional?
What is Decision Intelligence?
My experience of working in a data role within industry is predominantly as a data scientist in Decision Intelligence, which is the application of AI to the commercial decision making process. The core concept of Decision Intelligence, providing recommended actions that address specific business needs, is inherently outcome focused, requiring the assessment of four distinctive components to deliver a successful implementation of a DI solution:
- People: Stakeholder behaviors and judgments need to be carefully analyzed and considered to ensure the successful adoption of a DI solution.
- Processes: How a solution fits into an existing process, or an understanding of any organizational or process changes, have to be considered when designing a DI solution.
- Systems: How data feeds a DI application and the consumption of outputs to business users and systems.
- Data: Big Data is often associated with AI and DI solutions, but will vary across domains and industries. This can include typical transactional and customer datasets, to those which are much more nuanced, such as the production plan of a business. The scale, complexity and variety of data is only increasing exponentially, with data science at the heart of the disentangling…
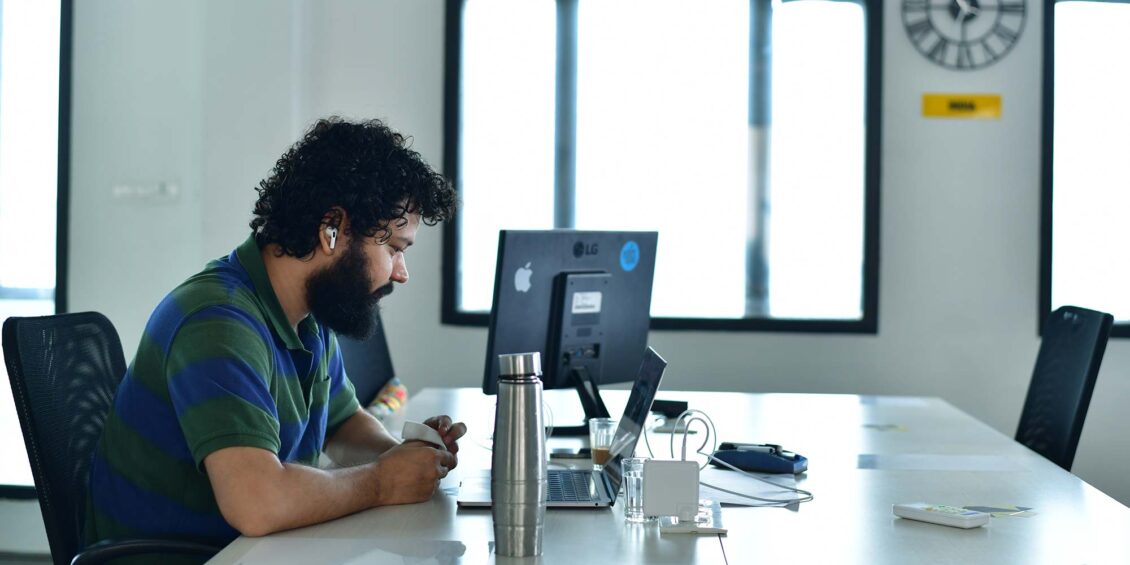
Decision Intelligence applications in retail
Within data science we build applications that target a range of decision points across the retail lifecycle, including omni-channel demand planning, optimal allocation and replenishment, pricing and markdown, and hyper-personalization of marketing and product recommendation systems. Here, we deep dive into one of our core applications we build, the personas we interact with, the systems and data to consider and the typical outputs that are generated.
Typical solution: Markdown Optimization
Markdown periods are ubiquitous across retailers; the winter and summer sale periods, and more recently ‘Black Friday’ are now planned for in every retailer’s calendar. However, deciding what the optimal price to set a product in a specific channel or store to maximize margins and ensure profitable sell-through is difficult, often requiring compromises that could lead to suboptimal results.
For example, you may have to set fixed prices for products across all stores rather than a more textured approach, taking into consideration the inventory levels and sell through rate of a particular store. A markdown optimization solution is able to analyze data at this granular level, providing store and channel specific recommendations for every product, maximizing key business metrics.
Who will you get to work with?
The personas we target when building markdown optimization applications are trading managers and planners in the merchandising team. They are responsible for selecting the products, spending the markdown budget, setting the markdown levels for each product and hitting the markdown KPIs (e.g. gross margin, sell through). These teams are very analytical, often building complex spreadsheets to support their markdown decision making, but often admit there is only so far you can go with a spreadsheet.
This sets the foundation for a productive relationship with a data scientist, enabling detailed and in-depth discussions over markdown recommendations that might emerge from the application. We have seen the surprised look on trading managers’ faces where a classic markdown strategy of ‘start shallow, then go deeper’, has lost out to an optimization strategy of ‘right price, first time’. However, convincing the analytical mind of a trading manager has its own challenges, so be prepared for heavy scrutiny over every output that gets produced.
What techniques will you use?
There are usually two components to a markdown application, the demand forecast and optimization. The forecast predicts the rate of sale of every product for each store/channel combination for every markdown discount, and the optimization decides what price to set to maximize the objective KPI.
We use state-of-the-art price elasticity and forecasting techniques to achieve the best prediction possible, which then feeds into the optimization. Business guardrails and constraints are set to ensure the outputs meet any business logic that is required.
What systems will it integrate with?
Our markdown applications typically involve two interfaces. The first is a system integration, which may be an API, or a file transfer, passing the markdown recommendations into an Enterprise Resource Planning (ERP) or other pricing tool. The second is a web application accessible within the Peak platform; both of which are built by data scientists on Peak.
This enables the end users to explore the recommendations, scenario plan (e.g. what happens if I want to maximize margin instead of revenue?) and make any changes. Any analysis that a merchandiser would typically perform is built into the applications as well, enabling the merchandiser to be more efficient in their role.
What results can you expect to see?
The markdown solutions that we have built at Peak have resulted in a three to five percentage point increase in margin for our customers for their markdown products, a boost in pricing efficiency and – in the case of one fashion retailer – up to 20 days of markdown planning saved. That had a huge impact on their team.
The greatest observation we have seen, though, has come from the change in mindset of our end users. Traditionally, retailers start shallow, with an average of 20% markdown, and go deeper nearer the end of the markdown period, with products marked down to more than 70%. This solution challenges this and proves that this approach is suboptimal, and that a ‘right price first time’ strategy of selecting a markdown that doesn’t change, will always win.
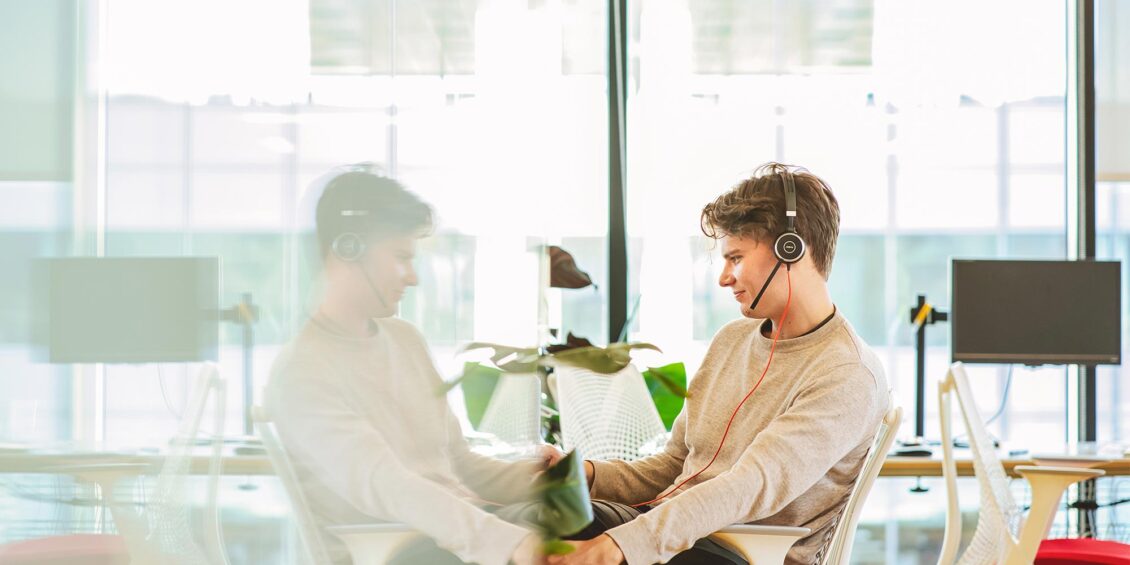
Decision Intelligence applications in manufacturing
Decision Intelligence is now becoming commonplace for many manufacturing businesses, using AI to optimize inventory, improve quality and minimize failures. This will drive greater operational efficiency, improve cash flow and minimize stock holding.
AI can support across many areas of manufacturing due to the abundance of high quality data available. At Peak, we build a number of solutions across the manufacturing supply chain, including deployment planning, stock keeping unit (SKU) rationalization, vehicle routing, customer order consolidation and pricing. However, one of our most popular solutions is Inventory Optimization.
Typical solution: Inventory Optimization
Having the right inventory in the right place at the right time is critical to running a successful business. When stock isn’t where it is needed, it leads to stockouts and missed sales opportunities; when businesses carry too much stock it makes planning, storage and transportation difficult and inefficient, resulting in capital held up in unwanted stock.
Inventory Optimization sets the correct target inventory levels at each location to balance the service, sales and availability gained from having extra stock with the holding costs and operational inefficiencies associated with holding too much stock. Quite simply, the output of this are the safety stock and reorder points required for each product. This can be set at different locations within your supply chain; for example, your manufacturing plants, warehouses and stores.
Who will you get to work with?
The supply or demand planner is responsible for managing inventory levels in a warehouse. They are tasked with setting reorder points for each product based on anticipated demand, balancing service levels for customers with the cost of holding inventory. They assess the financial capacity and inventory storage of the business, ensuring there is a steady flow of stock without over-capitalizing on inventory.
This may lead to changes in warehouse layout or financial forecasts. When tailoring our Inventory Optimization solution to a business, we work closely with the demand planner, identifying the guardrails that control the buying processes. This generally includes supplier constraints, storage capacity/cost, stock building and minimum stock levels.
What techniques will you use?
There are usually three components to an inventory optimization solution; a demand forecast, safety stock model and reorder point calculation. The demand forecast underpins the entire supply chain process, with drastic consequences if the uncertainty in the forecast isn’t managed correctly (for more info, see my colleague Tom’s blog on how to manage uncertainty in the supply chain.)
We then model our forecast uncertainty, i.e., the confidence we have in the accuracy of our forecast, to hedge against demand volatility. We use the output of this to generate our safety stock levels. Finally, we combine the safety stock levels with future orders and the forecast to determine the reorder point, taking into consideration the lead time of the product. The amount of the safety stock determines the service level of the product.
What systems will it integrate with?
The majority of businesses will use an ERP system to manage their inventory levels. Outputs are fed from our platform into ERP systems, either directly or via a proprietary system. Connections will either be via an API or bulk file transfer depending on the size and frequency of data transfer.
What results can you expect to see?
Using automated dynamic reorder points and safety stock results in optimal stock levels at SKU level granularity. Model outputs can be explored to assess the incremental increase in cost, for an increase in availability, allowing refined cost/benefit analyzes. Inventory Optimization solutions we have delivered at Peak have resulted in a 15-20% reduction in stock and a 2% increase in availability.
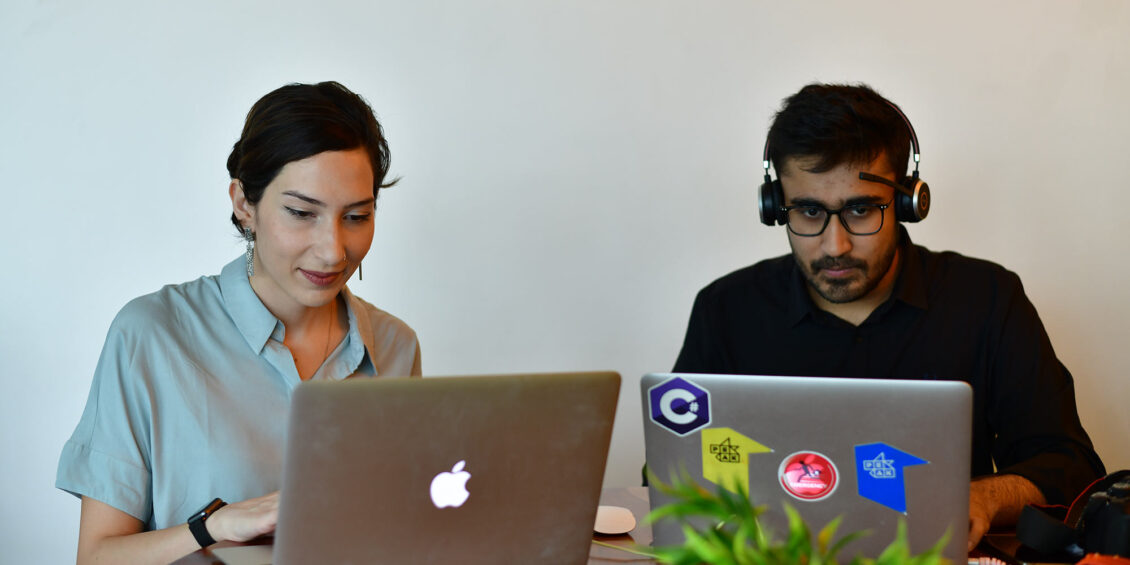
Decision Intelligence applications in consumer packaged goods (CPG)
Staying ahead of the game and maintaining household relevancy is becoming harder as consumer preferences constantly shift. As businesses aim to deliver omni-channel experiences, leading to greater complexity in supply chains, streamlining processes is becoming harder. Yet with core business functions handling large volumes of data, Decision Intelligence has the potential to impact many areas of the supply chain. These include trade promotion optimization, demand planning, pricing, personalized consumer engagement and risk management. Here, we discuss one of the major components of a CPG supply chain: its logistics network. Let’s take a look at how data science and DI can optimize distribution resource planning.
Typical solution: Distribution resource planning (DRP)
To optimize a business’ network of distribution centers is complex and challenging. To ensure a business is able to meet consumer demand, products need to be in the right place at the right time in the network. Predicting demand at every location is inherently complex, but deciding what decisions to make off the back of them, with the current state of your network, is even harder.
This solution provides a way of automating these otherwise time-consuming processes, aiming to maximize service levels and minimize the cost of ordering, transporting and holding goods. The solution decides what quantities of products to move between each location (distribution center) and the most efficient method of transporting the goods (e.g. the number of trucks required to fulfill demand.)
Who will you get to work with?
The distribution resource planner lives in the supply team, planning and coordinating the product distribution activities for the business. They manage materials in warehouses, determine shortages and excesses of supplies and arrange supplies for distribution. They work with a number of different teams including supply chain, warehouse, suppliers, IT and logistics to ensure distribution orders are processed correctly. A data scientist will work closely with the planner to identify business guardrails and constraints that need to be implemented and assess the viability of the solution.
What techniques will you use?
Our DRP solution takes our inventory optimization solution one step further, not only deciding how much stock to hold at each location, but how best to distribute that stock across the network.
This takes into consideration current inventory levels, products in transit, back orders, the production plan and logistical constraints in the network. A multitude of optimization methods may be applied to solve this problem, a few examples include mixed integer programming and simulated annealing.
What systems will it integrate with?
Similar to Inventory Optimization the outputs will feed into an ERP system, either directly or via another Business system. Suggested orders can be amended and accepted through an interface on Peak before being submitted for execution. Detailed insights into stock forecasts, production forecasts and consumer demand on Peak enable greater visibility and oversight of stock management.
What results can I expect to see?
A DRP solution will minimize the redistribution of stock and maximize availability, resulting in optimal service levels for products. This will reduce the costs of ordering and help minimize a businesses carbon footprint, supporting sustainability initiatives that are becoming increasingly common across businesses. Peak’s DRP solution has redacted truck journeys and CO2 emissions by 4% over a nine month period.
These are just some of the types of applications data scientists build in a number of industries and the benefits they provide. The future will certainly bring even more opportunities, especially as the line between AI businesses and industry business fades; pretty soon all organizations will have adopted DI in some form or another. It will just be down to you to decide what kind of data scientist you want to be!
Looking for a new challenge in data science?
We're on the hunt for more brilliant minds to join our team as we continue to scale. Take a look at some of our current opportunities!
More from the Peak data science team
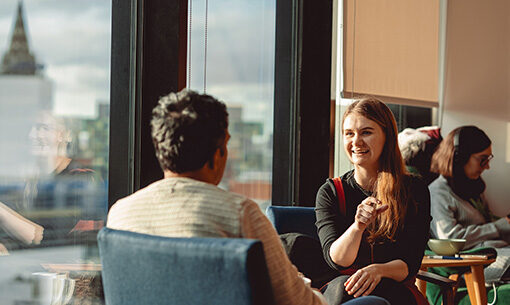