Just a heads up, AI does lead scoring better than your sales team
By Richard Potter on December 7, 2017Have you ever looked at the menu in a restaurant and thought, “these dishes all sound nice, how will I decide which to have? I wonder how fresh the fish is? And where the lamb is sourced from? And how well the chef can cook a rare steak?”
When you’re sat in a restaurant struggling to choose between dishes, you have to make an educated guess about what factors will most affect the quality of each meal. It’s only when the waiter serves your order, though, that you’ll know if your instincts were right, or if you should have taken a chance on the salmon instead.
In essence, you’re basing the decision about how to choose your meal on incomplete information. If you’re an experienced food orderer, you might choose factors that are pretty good measures of what you should go for. If so, enjoy the steak – you’ve earned it. Don’t be under any illusions though – those same factors might not work as well next time you’re ordering a meal.
This process of choosing between menu items in a restaurant is a lot like traditional lead scoring (*SEGUE KLAXON*)! Companies that need to distinguish between the quality of their leads, so that they can prioritise the most important ones, have typically had their sales teams flag up the factors they think are most important in getting leads over the line and attribute points relative to how important they think they are.
The problem is that, in spite of all of the sales experience and expertise that may be at play, there’s no guaranteeing your sales guys will choose the best factors. Or to know whether they have. Or that they’ll attribute the right points to the factors that they’ve chosen. Or be sure that they have. Suffice to say, traditional lead scoring is a recipe for uncertainty.
Artificial intelligence lead scoring, though. Well that’s some Michelin-starred lead scoring. The reason for this is that it leaves nothing to chance. Instead of us mere humans trying to determine what factors might best indicate the chance of a lead becoming a customer, AI can find the sometimes-hidden patterns and trends within data that unequivocally do.
To do this, it just needs to be set to work, scouring all of a company’s customers to find out what common traits they have. Then, it can trawl the company’s sales pipeline to find out which of the potential clients share those traits and to what extent. A score can then be applied to each lead in the company’s CRM to help salespeople prioritise them.
Regit, formerly Motoring.co.uk, is an example of a company with which we’ve employed this approach ourselves. The firm generates leads for companies in the automotive industry by providing a way for people to book test drives, buy and sell cars, request brochures and suchlike. The issue was that they treated their leads the same, regardless. So, with lead scoring they can now prioritise which leads to focus on and how, and this then resulted in the revenue jump.
We pulled together data from Regit’s website and marketing systems, as well as from third-party datasets, such as from the DVLA, and applied some categorical machine learning models (as you do) to give predictions about the likelihood of users changing car. Lead scores were then pushed into Regit’s CRM system. Regit saw a 27% increase in sales and reduced operational costs by up to 35% by simply staffing the call-centre at the times of day most likely to result in a sale to a user or customer. I think we can all agree, they are some tasty figures.
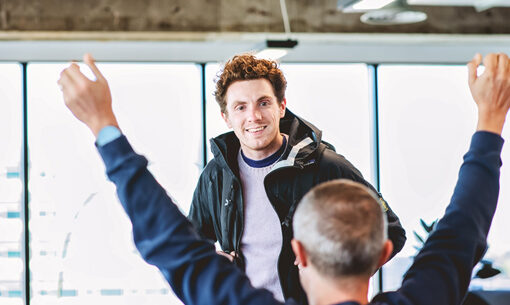