How AI can optimize customer segmentation: a guide
By Jon Taylor on February 6, 2022 - 10 Minute ReadHave you ever had an experience buying a product from a brand…and you felt like the company was reading your mind? ?
Before you made the purchase, the company probably knew a lot about you — what products you’d been looking at, where you live, how old you are and much, much more.
And all of this data and information was used to add you to a customer segment that targeted your likes and interests perfectly.
Of course, customer segmentation isn’t new and is one of the key components of any successful marketing machine. But thanks to the rise of artificial intelligence (AI) customer segmentation, it’s getting smarter, more accurate and creating better customer experiences. It’s good for companies, too. By analyzing large amounts of customer data, brands can understand what products their customers really want.
This article will explain the importance of AI customer segmentation and how you can use it to connect with your customers.
Table of contents
- What is customer segmentation?
- AI customer segmentation is changing the game
- The four types of customer segmentation AI explained
- Three real-life use cases of customer segmentation using AI
Let’s get started.
What is customer segmentation?
Customer segmentation is when customers are divided into smaller groups based on similar likes, characteristics and demographics.
By segmenting customers based on certain criteria, businesses are able to create more effective marketing strategies and tailor their products or services to the specific needs of each group.
But traditional customer segmentation, done by a human, has pitfalls. It’s a very time-consuming process and involves lots of digging into collected data to figure out which customers should be placed into specific segments. It’s also prone to:
Limited data and insights
Traditional customer segmentation relies on data silos and spreadsheets that need to be manually updated and analyzed. As customer bases grow, it’s hard to keep the data relevant and gather enough details to make insights useful for segmentation.
Human error
Customer data was traditionally updated manually by team members — a slow, manual process that’s prone to human error. Analysts may make inaccurate assumptions or import data incorrectly, leading to flawed customer segmentation.
Problems with scalability
Human data collection and customer segmentation is doable with a small customer base. But as a business starts to grow, keeping customer data accurate can become impossible. Manual customer segmentation is so time-consuming that it’s just not feasible for large customer bases.
Traditional customer segmentation may be flawed, but using AI and machine learning can help solve most of the problems around manual data entry and scalability.
AI customer segmentation is changing the game
AI customer segmentation fills the traditional customer segmentation gap by automatically splitting customers based on certain characteristics or behaviors.
But that’s not what makes it so effective. It’s cutting out the risk of human error and limited data and replacing it with artificial intelligence techniques that learn about customers. The more data the AI has access to, the better it understands customers and what they need.
For example, a financial institution is launching a new product targeted at high-income customers. It can use AI to find customers with similar savings patterns and incomes to create specific marketing campaigns that will appeal to their investment goals. Without AI, a task like this would take hours, and a customer’s spending patterns and behaviors could easily be missed.
AI customer segmentation can also be used to identify customers who are at risk of churning so you can focus on retention.
It can spot characteristics or behaviors linked to customers who have churned and flag current customers who are likely to leave. Armed with this information, you can reach out to high-risk customers and offer support to keep them around.
AI customer segmentation can also be used to identify customers who are at risk of churning so you can focus on retention.
The four types of customer segmentation AI explained
There are several ways to segment customers using AI by tracking data like buying behavior and demographics.
Here are the four most important types of customer segmentation in AI ?
1. Demographics
Using this type of segmentation, customers are divided into groups based on their age, gender, education, annual income, marital status and occupation.
Example: An online clothing store can use demographics to segment customers and create targeted email campaigns. For example, Gen Z women who earn over $100k and work in corporate offices can be targeted with high-end garments on their chosen social channels. Here’s how Frankie Shop targets a customer segment like this on Instagram:
Image source: @thefrankieshop on Instagram
2. Geographic
Customers are grouped into segments based on their specific location, like their country, city or suburb.
Example: A fast food chain might use geographic segmentation for advertising different dishes based on cultural differences and preferences. Here’s how McDonald’s segments customers and targets them with different dishes depending on their location:
3. Behavior
Behavior segmentation splits customers into groups based on their previous purchase history, brand loyalty, spending habits and overall lifetime value.
Example: A financial institution might use this segmentation technique to split customers based on risk tolerance, income and spending habits. It can create two segments of customers: those who primarily use their debit card and those who prefer to use their credit card. On the back of this, the business can target these customer segments individually with different products, like lower interest rate credit cards, that may interest them.
4. Psychographics
The last type of customer segmentation is psychographics, which divides customers into groups based on personality, lifestyle, interests, morals and values.
Example: Brands like Patagonia and Ben & Jerry’s use psychographics to appeal to customers’ interest in social and environmental issues. Targeting these specific segments helps build trust in their customer bases and prove to them that the brand is invested in the same issues they are.
Image source: Ben and Jerry’s via Bthechange Medium
Some businesses may even merge different segmentation techniques together to get a better understanding of its customers. For example, an ecommerce store can use AI customer segmentation to optimize:
Product recommendations
Customer preferences and interests could be segmented through machine learning by analyzing shopping history and browsing habits. This data can help shape personalized product recommendations that can be sent to specific customer segmentations to boost sales.
Pricing
AI can analyze customer data and patterns in buying behavior to calculate optimal price points for different products or services. This gives a brand the best chance at keeping prices competitive without losing customers to competitors.
To get a better idea of how these customer segmentation techniques work, let’s check out some real-life examples.
Three real-life use cases of customer segmentation using AI
Have you purchased something from a popular brand in the last year or two?
If the answer is yes, chances are that your purchase was, in some way, influenced by AI customer segmentation. Brands now use data about our likes, dislikes, buying behavior and location to deliver hyper-targeted and hyper-personalized experiences.
Here are three examples of real-life brands using AI customer segmentation to get in their customer’s heads ?
1. PepsiCo uses AI to improve product and customer experience
PepsiCo was an early adopter of AI technology, using it to personalize marketing and product development as well as overhaul its supply chain.
Let’s start with how the brand uses AI to create new products. It uses a tool called Tastewise to track what customers are eating and drinking with PepsiCo products using algorithms. The tool monitors billions of touchpoints across the internet, like social media posts, recipes and online menus to see how and when customers are consuming PepsiCo products.
This data is then fed to PepsiCo’s product development team to help them create food and drinks that customers are asking for. Using this data, the company launched a snack range called Off The Beaten Track.
The main ingredient? Seaweed.
“If you would’ve asked consumers, ‘tell me what your favorite flavors are and let us know what you think would be a great flavor for this brand,’ nobody would have ever come up with seaweed,” the company’s chief consumer insights and analytics officer, Stephan Gans, told VentureBeat.
“People don’t associate that typically with a specialty snack from a brand. But because of the kind of listening and the outside-in work that we did, we were able to figure that out through the AI that’s embedded in that tool,” he said.
PepsiCo even uses AI to stock individual stores with different products based on what its customers buy. It collects data from stores so that PepsiCo can better predict the snacks that individual stores need to stock to better meet the shopping needs of consumers.
Data is collected and stored in the company’s application called Store DNA. The application analyzes individual customer and store data to see what’s selling best. Then, the app tells PepsiCo’s field associates the top three product actions to take at each location, like stocking up on a particular item.
How PepsiCo collects data using Azure architecture. Image source: Microsoft
“Now we can use data to tell our field associates which products will have the biggest impact that week, what the associates need to watch out for, and what they can do to make sure they really meet customer expectations — things they might not have been aware of before.”
“They can better capitalize on sales opportunities thanks to insights drawn via machine learning from our vast data warehouses. Due to the scale and scope of the data, we wouldn’t have been able to even contemplate doing this sort of work until recently, with tools like Azure Machine Learning and its MLOps capabilities.”
— Michael Cleavinger, PepsiCo’s Senior Director of Shopper Insights Data Science and Advanced Analytics
2. ASOS uses AI to recommend the right products
E-commerce giant ASOS adds 5,000 new product lines to its online store every week.
So, how does it ensure customers know about them and get accurate product recommendations to boost sales?
Easy. The company has invested in AI customer segmentation technology to ensure shoppers have a great experience in their store. In 2018, the store launched its “Fit Assistant” feature to help customers always choose the right size clothing. The tool asks shoppers for their height, weight, age, hip and waist appearances, preferred fit (tight, average or loose), measurements and their current size in any popular brands they wear.
On the back of this information, the Fit Assistant AI tool will recommend a product size and show the percentage of fellow ASOS shoppers who had similar results and the size they ended up purchasing. The more customers use the Fit Assistant, the more data it has to feed on to become more accurate.
This is just one example of ASOS using smart marketing to learn more about its customers. It then uses all of this data to create a better shopping experience using personalized product recommendations. Thanks to machine learning algorithms, ASOS analyzes every customer’s purchase history and browsing patterns and uses this data to create personalized recommendations for specific products.
It does this using “product clusters”, which joins similar products or buying preferences together before showing them to the customer:
Each group contains 36 of these clusters and using machine learning, these products are recommended at different touchpoints throughout the customer’s journey.
But what makes ASOS’s use of AI customer segmentation really stand out is how it uses it to spot valuable customers. The company’s deep learning neural network can predict the risk of a customer churning using demographics, purchase history and a combination of churn classifications:
This information is then used to offer different products and promotions to high-value customers that are at risk of churning to keep them around.
And to top it off, ASOS also uses AI to predict when a product will run low and ensure it’s restocked. This algorithm analyzes sales data, trends, customer behavior and current stock levels to predict which products are at risk of selling out and need to be replenished.
This data means the risk of a customer ordering a product online and ASOS not being able to deliver the goods stays very low.
3. Uber uses AI to ensure customers always have a ride
Uber uses AI to segment its customers based on their location, transportation needs and rider preferences.
The company’s app is a data goldmine: customers order lifts, give feedback and add their locations into it. Uber then collects and analyzes data like the customer’s ride history, vehicle preference, frequent pickup locations and frequent destinations to spot patterns.
These patterns are then used to:
Create predictions
Is a customer usually ordering rides from Battery Park on a Friday evening between 22:00 and 01:00? These patterns help Uber predict when a customer will likely need a ride. This data then feeds into Uber’s algorithm and helps it meet supply and demand to make sure the customer always has a ride available.
Cluster customers
Customers are clustered together using Uber’s algorithm based on past riding behavior, trip length, location and average pickup times.
Personalized ride options
Uber uses machine learning to analyze customer preferences and behaviors to recommend the best ride option for each user. For example, it will suggest an UberPool ride for a customer who has consistently taken shared rides in the past.
Uber has even created an AI model to segment customers based on location so they get real-time estimated arrival times for their rides. The customer’s ride request is routed through the model’s uRoute service before it makes requests to the routing engine to create route-lines and ETAs.
For Uber, the ultimate end goal is to create a better customer experience and think of every rider’s needs before they do.
Thanks to AI and machine learning, Uber can gather and analyze huge amounts of data, fast. Because the company has millions of frequent customers, making predictions and analyzing this data is impossible. Using AI allows Uber to create more accurate segments of customers and personalize every service to the specific needs of every rider.
Introducing headless segmentation
Headless segmentation is a new approach to marketing that we’re currently pioneering at Peak. This empowers our customers to create more targeted, sophisticated customer segments than ever before — enabling them to have truly one-to-one conversations with their audience, across every single touchpoint.
What do we mean by headless segmentation? We mean segmentation that isn’t bound to a specific system, channel or silo — meaning you can leverage information from multiple sources to paint a more holistic picture of your customers. When you combine this game-changing approach to segmentation with the power of AI, you can not only build meaningful customer segments based on attributes that may otherwise have been missed, but you can also predict what these segments are most likely to do next!
You can find out more about this exciting new concept by watching our headless segmentation webinar on demand.
Discover the future of customer segmentation
Learn how headless segmentation can help you create meaningful cohorts of customers. Watch the webinar on demand now.
Are you ready to take advantage of AI customer segmentation?
AI customer segmentation can be used for just about everything, from better product recommendations to spotting customers who are at risk of churning.
Not only does it fix a lot of flaws in traditional customer segmentation, like limited scalability and human error, but it turns customer data into a powerful marketing tool. AI can uncover customer trends that would be impossible for humans to spot, like certain buying behaviors or product trends.
With the right tools, you can create AI-powered customer segmentations that supercharge revenue and improve customer experience.
More on customer segmentation
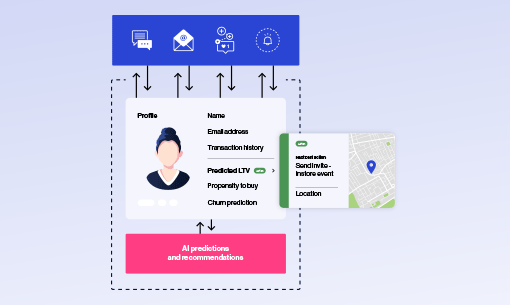
Headless Segmentation: The only way to win with your customers
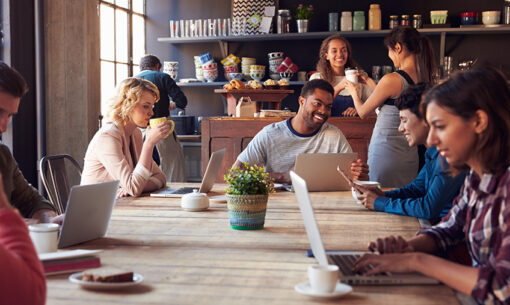