Data science graduate diary: I fell in love with manufacturing data science!
By Nikolas Heinloth on March 25, 2022Hello! Do you ever feel like a little kid in a candy store, struggling to decide on which area of the vast field of data science to try first?
Well, let me help you out by sharing my experiences of being part of the Consumer Packaged Goods (CPG) & Manufacturing team at Peak!
Let me introduce myself first. I’m Nikolas, and I joined Peak as a Graduate Data Scientist six months ago. The core idea of the graduate scheme at Peak is to get a guided tour through the metaphorical candy store that is our data science department. Us grads get to sample the entire product offering: Insights, Peak Labs, as well as Retail and CPG & Manufacturing which are captured within the wider Operations team. While I will focus on the last mentioned, you can get first-hand insights into the other teams in the blog posts of my fellow grads Amy, Ben, Kira and Chrys.
What typically characterizes an operational data science project in manufacturing?
Within this area we’re mostly talking about solutions that assist businesses in getting products from the warehouse to the customer. This covers the entirety of a traditional supply chain: information flows, transportation, shipping, warehouse costs, stock levels etc.
What problems do we cover?
At Peak, we build a number of solutions across the manufacturing supply chain, including deployment planning, stock keeping unit (SKU) rationalization, production scheduling, vehicle routing planning and pricing, to name just a few. One of our most used solutions is inventory optimization.
I was lucky enough to be allocated to a project working with a global adhesives and chemicals manufacturer. The customer had a lot of capital and storage space tied up in inventory for relatively slow selling SKUs (aka products), but was unable to deliver popular items to their customers during times of high demand.
What is inventory optimization?
Inventory optimization looks at balancing capital investment constraints or objectives, i.e. the cost of holding stock and service-level goals over a large assortment of SKUs. The tricky part of the solution is adequately taking demand and supply volatility into account.
Who did I work with?
The team I joined is relatively large, with three senior data scientists working on the project, complemented by one customer success manager (CSM). The CSM’s role within the project team is focused around project management, prioritizing tasks, aligning with stakeholders and negotiating the customer’s wishes with deadlines and associated effort for the data scientists. Getting the opportunity to work closely with that many people was super helpful for a number of reasons:
- There was always someone to turn to with any questions
- I had the opportunity to learn by engaging with all the novel aspects of a fully fledged data science project and growing into my role within the team. Mutual learning, by the way, is not reserved for new joiners or graduates; it represents a core value at Peak that everyone lives and breathes
- Every team member brings their own unique skill set to the table. All of which come in handy at different stages of a project’s life cycle and set the team up for success
Mutual learning is not reserved for new joiners or graduates; it represents a core value at Peak that everyone lives and breathes.
Nikolas Heinloth
Graduate Data Scientist at Peak
What does a typical project lifecycle look like?
The data science journey of the project starts after the commercial team have worked their magic and a rough scope of the project has been defined. With a clear objective in mind and the data feeds set up, we get to work!
Ideation and solution outlining
We start off with one of the most exciting elements of the project: the ideation sessions. Corner office with plenty of window space? Check. Big whiteboard? Check. Large stack of post-its? Check.
Time to sketch out a roadmap of how we are planning to achieve the set objectives as efficiently as possible. While such bundled brainpower may initially feel intimidating, every opinion and every approach is valued and discussed. This made me feel immediately involved and respected in the discussions.
Data cleansing
Once we’ve translated the data questions into an actionable roadmap and have access to the raw data, the data needs processing before any further analysis can take place. This part of the project can feel a bit tedious and long-winded at times, especially if the customer relationship management (CRM) system is a relic out of the pre-Pac-Man era. To get our ‘AI-ready’ datasets, we implement data engineering pipelines which help us process and clean the data.
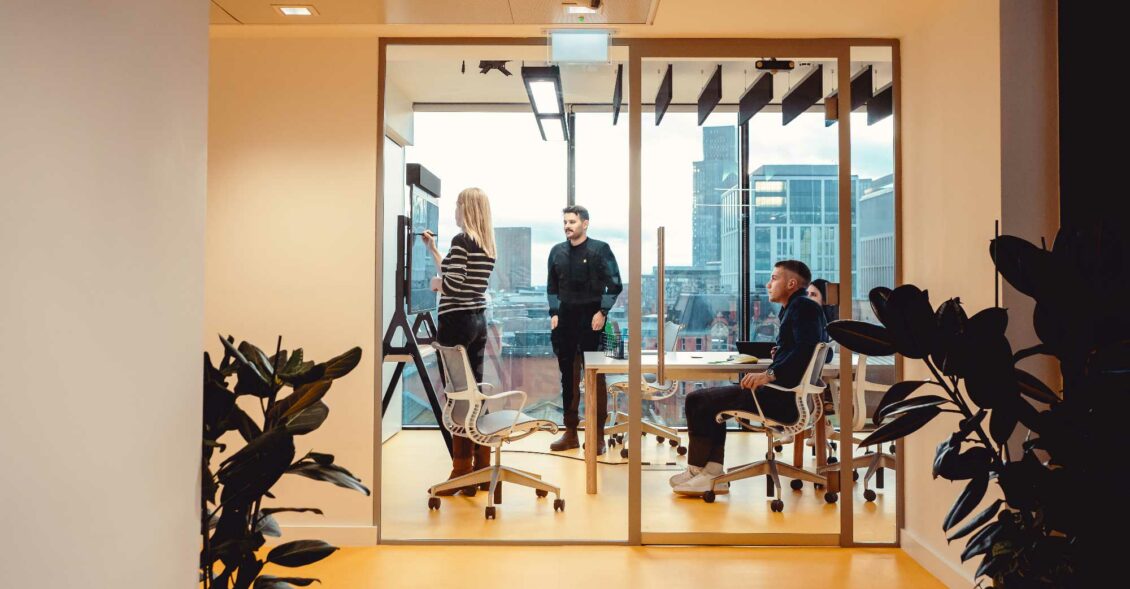
Exploratory data analysis
Data analysis is where you can leverage all of the data science tools at your disposal to crunch the data and find every insight you can. It’s that analysis that will set you up for the subsequent modeling stage of the solution and often delivers already-valuable insights to the customer.
In my case, those insights were entered around performing unit tests, analyzing demand and supply patterns, or performing analysis on lead times. With regards to the language and methods you use, you are generally granted total freedom – but you can, of course, always turn to a more experienced team member for a bit of guidance should you need it.
Get coding
Here comes the fancy part of the project…the AI magic we’ve all been waiting for! If I had to choose one learning from my first rotations then it’d be to follow a crawl, walk then run approach. Start off with a simple model, ensure that the logic works correctly and that its outputs can be actioned within the business, feed it back to the customer and then potentially reiterate on it.
It sounds like double the work but, trust me, it will not only deliver faster value to the customer, but it will also make your life a whole lot easier. As a fast growing company, we try to keep in mind not only the actual project success, but also learnings and efficiency gains for future applications. We could, for example, partly rely on tools and packages built in-house to speed up our time-to-value process.
Communicate results
Once the model is working, and the outputs are valid…we’re done, right? Far from it, actually! Proper communication of the results will mean the difference between action and inaction on your solution. This can mean a number of different things.
In our project, it involved building a dashboard surfacing purchase order recommendations on a SKU level. Putting yourself in the shoes of the customer and crafting a presentation or user interface that ties your data with their knowledge is easier said than done. I found this stage of the project to be a very rewarding experience. Ultimately, it’s the final piece that’ll bring the solution to life for the end user.
Listen to what’s wrong and re-iterate
Just as important as communicating your results to the customer is taking their feedback on board. Revising the solution you build is essential to ensure user adoption and long-term project success. Your model can be the best of its kind, backed up by a stunning dashboard – but if no-one uses its outputs, it’s not really worth it.
Key takeaways
Why did I love this rotation?
I’ve now told you a whole lot about my experience in the manufacturing data science team, and given you a glimpse at the different facets that a customer project brings with it. But the best bit?! That was the great people I had the pleasure of working with, and the variety within the project that made me enjoy my time so much.
Variety manifests itself both in terms of different problems you might be working on, but also the full spectrum of data science skills. I had the chance to develop the project from a sketch scribbled on a whiteboard up until the final presentation and a live demonstration to the customer. This multitude of tasks coupled with a good balance of theoretical work, customer interactions and actual hands-on coding meant that there was always an area of potential for further personal development and growth.
What am I now more mindful of?
Is it a bit of a biased view through rose-tinted glasses? Yes, probably. As with everything in life, the benefits I’ve been raving about have also come with a few challenges to keep in mind. Beware of shifting workload, ad hoc requests and deadlines. Correct prioritization of tasks and focusing on the important bits is paramount to managing your workload and keeping you from feeling overwhelmed. Is it something for everybody? Probably not. But you never know until you try!
Interested in a career in data science?
Take a look at our current opportunities via the link below!
More from our data science grads!
Check out what some of other members of the current DS grad scheme cohort have been up to so far!
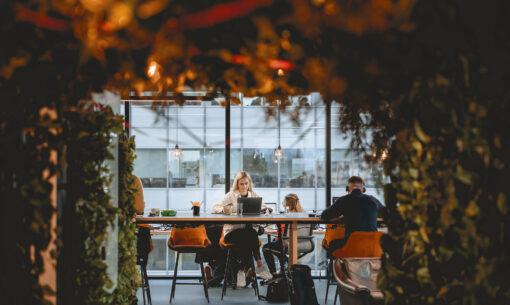
Why I joined Peak as a Data Science Graduate
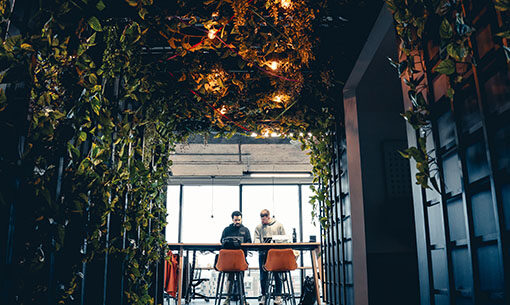