A simple guide to multimodal machine learning
By Jon Taylor on July 24, 2022 - 10 Minute ReadWe understand what it means when we look at an image or read text. Up until recently, AI didn't have the same skills. But the invention of new technology, multimodal machine learning, is starting to change that.
Researchers from billion-dollar companies like Google and Microsoft have spent years building these tools, and the results are impressive. A multimodal AI system can not only interpret images, but it can also create them using simple text commands. Unlike previous AI systems, Multimodal AI can process several datasets simultaneously to eliminate bias and create an accurate depiction of input data.
For years, Multimodal AI has been sidelined by computer scientists and studies. But now, companies are investing in the technology to improve customer experience, create original imagery and speed up video processing.
This article will take a deep dive into how multimodal machine learning works, how it’s used and what it means for the future of business.
We’ll cover…
- What is multimodal machine learning?
- What is multimodal AI, and how is it used?
- Three real-life use cases for multimodal machine learning and AI
What is multimodal machine learning?
At a high level, machine learning is an application of AI — one that is increasingly being used in a business setting. ML allows systems to be able to learn automatically, improve from experience, and get smarter over time, without being explicitly programmed in a certain way.
Multimodal machine learning (MMML) combines data like text, speech and images with linguistic, acoustic and visual messages to achieve higher performance.
Using multiple data and processing algorithms, MMML can react to visual cues and actions and combine them to extract knowledge. For example, MMML can use Natural Language Processing (NLP) to extract and understand data, relate it to keywords and understand semantics. Combined with speech processing like trigger word detection and language model extraction, it allows the technology to process data and better understand certain situations.
However, some challenges come along with MMML. AI is limited in finding a common language to communicate and translate data so it can be used. There are also limitations on how MMML transfers knowledge between systems and how it associates data with predictions. And it’s also crucial to understand the nuances and differences between multimodal learning and multimodal AI…
Using multiple data and processing algorithms, multimodal machine learning (MMML) can react to visual cues and actions and combine them to extract knowledge.
What is multimodal AI, and how is it used?
Multimodal AI combines data like images, text and speech with algorithms to create predictions and outcomes.
Think of multimodal AI as a human brain that can process several things at once. You may see a cup of liquid that looks like coffee, but you’ll only be sure after tasting it. The color and smell help narrow down that it’s a cup of coffee, but the taste and temperature will confirm it. This is how multimodal AI works: piecing together different bits of information from separate data points to form an accurate conclusion.
It’s also quite different from traditional ‘unimodal’ AI systems. While multimodal AI can be adapted to different inputs and outputs, unimodal AI is designed to perform one specific task, like processing images or text. The problem with traditional AI is the way it’s designed lends itself to bias; it only has one data point to work from, and the lack of context or backup information can end in it drawing inaccurate conclusions.
Multimodal AI overcomes this by cross-referencing data points and ultimately using them for accurate confirmations. But what exactly does this look like? ?
Multimodal AI could soon replace chatbots
Meta (or Facebook to you and me!) is currently leading the way with multimodal AI tech that can emulate a human’s reaction to queries.
Here’s how its tech, named FLAVA, works. Suppose a customer messages it and explains they want to buy a new pair of black jeans in different styles and materials. In that case, the assistant can reply with different images and information on each product. It does this using a series of prompts that pair the customer’s text with language processing and reasoning:
The technology was trained using images in over 35 different languages, so it can also create captions using masked image modeling (MIM) and multimodal encoders. Here’s an example:
The image of a cat is turned into a caption over several steps. First, the image is embedded into FLAVA’s transformer model, which extracts a list of hidden state vectors and classification tokens. Next, the text encoder embeds the text inputs into vector lists and eventually turns them into tokens. The final result is a caption that describes the image and the cat’s actions and thoughts.
Microsoft is developing similar technology that translates captions and text into images. In this example, the multimodal technology is fed English and non-English text to make sense of the images:
The model then projects an image representation sequence based on the projected visual feature vector and spatial embedding vector of each region in the image.
NUWA, another Microsoft-based project working with Peking University, is taking this type of technology one step further and researching if it can create new images and predict sequences from text. The researchers trained the model using videos, text and images. When it’s given a prompt, it predicts what image or video will be shown next or fill it in if the sequence is empty.
Multimodal AI is revolutionizing text translation
Multimodal AI isn’t being used for simple translations. Japanese researchers tested multimodal AI to translate manga comics and detect character gender. The study used scene grouping, text ordering and semantic extraction to translate information into text and context from comic scenes using speech bubbles. The end result is the technology can translate Japanese speech bubbles into various languages like Chinese and English, instantly and automatically.
Three real-life use cases for multimodal machine learning and AI
While translating Manga into English and turning text about dogs into images is interesting, it’s hard to see the connection for businesses that want to invest in multimodal AI and reap any commercial benefits.
The good news, though, is that multimodal AI can help automate manual tasks and eliminate bias for companies using data, text and images frequently. Here are three real-life use cases ?
1. Microsoft’s DALL·E
If you’ve heard of a multimodal AI tool before, it’s likely to be Microsoft’s DALL·E and DALL·E 2. The New York Times describes DALL·E 2 as the AI that “draws anything at your command.” And this description is largely true. Journalists took it for a test drive and asked it to create teapots in the shape of an avocado. Here’s what they got:
The technology uses natural language processing and applies text to image pairs using tokens. It does this by scouring millions of images in seconds and looking for patterns described in the text instructions, then creating an image on its research.
For brands that need original images for products or marketing campaigns, it’s easy to see how the technology could save hours of time (and money) hiring a designer or illustrator.
And similar technology is being trialed by The Allen Institute, where researchers are testing how well AI can analyze images in text alongside audio. After analyzing millions of YouTube videos, the tool could pinpoint moments in certain movies or television shows, like a door shutting or a dog barking. This technology could significantly reduce editing time if your company regularly works with video.
2. Renesas Electronics and Syntiant’s voice control
Have you ever told your RoboVac to stop cleaning or issued instructions to a security camera? If the answer is yes, the systems may be embedded with AI-based IoT systems, created by Renesas Electronics and Syntiant. The technology aims to give companies a quicker and easier way to integrate AI systems into existing infrastructure, like video conferencing and smart devices.
As the technology uses image and voice processing along with vision AI, it can spot anomalies in real-life situations. For example, if operator behavior in a warehouse is detected as being unsafe or not working to full capacity, it can send out a warning. Warehouse operators can also use phones and tablets embedded with multimodal voice interfaces to send instructions to machinery and improve productivity without touching them.
3. United Airlines’ customer service AI
Some of the biggest companies on earth, like United Airlines, are now investing in multimodal AI to improve customer experience. The company recently teamed up with NLX to roll out a multimodal AI tool so customers can interact with its voice assistant and change travel plans by chatting with it on the phone.
According to United, the AI-powered assistant can tell customers if a flight is running on time and answer queries relating to wheelchairs or accessibility needs. It can also upgrade their seats, quote flight prices and help with check-in without help. If the customer has a more complex query, the assistant will connect them with a human agent. By automating common and simple requests, United Airlines now leaves 70% of all customer inquiries to the AI-powered assistant.
Multimodal machine learning is the next step for tech-savvy companies
Multimodal technology is changing how businesses operate — even if you don’t know you’re using it. Forbes has now labeled Multimodal AI tools as the logical next step for companies that use video applications and images, and it’s easy to see why. Simple tasks like customer service, warehouse productivity and translation are being automated thanks to Multimodal’s ability to synthesize multiple pieces of information at once.
For businesses, the opportunity to create a better customer experience while automating manual tasks is an exciting next step. The question is, are you going to take it?
Transform the customer experience with AI
Know your customers like never before and provide them with highly personalized experiences. Discover how with our Customer Intelligence AI applications.
More from Peak
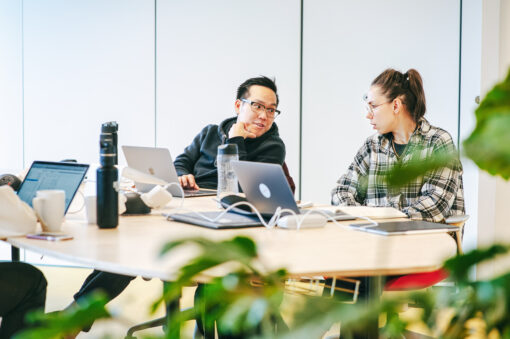